Author: Simone Maimon
Editors: Kacey Ye, Hwi-On Lee
Artist: Emily Hu
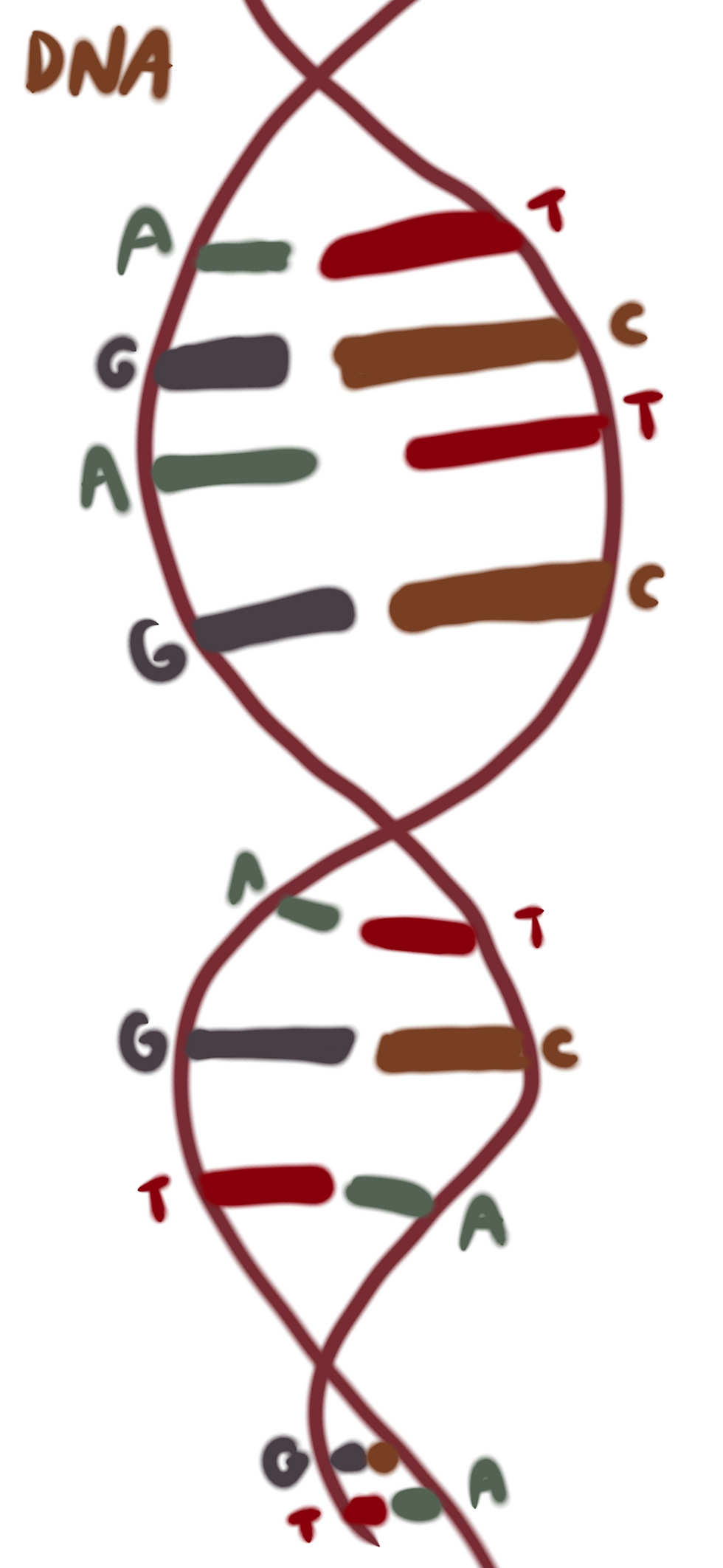
From the simplest prokaryotes to the most complex eukaryotes, proteins have always been essential to everything biological, facilitating replication, catalyzing reactions, and organizing the cell. At its core, proteins are polymers of amino acids linked together like a chain. Although a linear sequence of building blocks may seem easy to model on a computer, until recently, efficiently determining protein structure has been challenging for scientists. To better understand the complexity of proteins, biochemists have broken down protein structure into four classifications: primary, secondary, tertiary, and quaternary.
The primary structure of a protein is composed of a sequence of amino acids. In genetic material, the four bases that make up these amino acids are Adenine (A), Thymine (T) or Uracil (U), Guanine (G), and Cytosine (C). The bases are listed in order so they can be “read” by proteins to get instructions for protein formation. Every group of three letters signifies which of the twenty amino acids must be added to the growing chain. Bonded amino acids can rotate around the carbon-carbon and carbon-nitrogen bond. However, since the amino acid’s molecule prevents overlap, this causes limitations in the rotation angles. A Ramachandran plot can be used to graph the angles the two bonds can form relative to the central atom to see which angles are plausible within the amino acid.

Two areas on a Ramachandran plot represent common pairs of bond angles for the carbon-carbon and carbon-nitrogen double bond. These regions are associated with the amino acids folding into two structures: alpha helices and beta sheets. Known as the protein’s secondary structure, these structures are formed because of the hydrogen bonds between the nitrogen-bound hydrogen and the carbon double-bonded oxygen in different amino acids. Hydrogen bonds are not actual bonds between atoms but rather attractive interactions that are thermodynamically favorable, releasing energy when they are formed.

The tertiary structure is the combination of these various motifs put together. There are a few commonly found motifs consisting of multiple secondary structures, such as the beta-alpha-beta motif. The quaternary structure describes how many proteins interact to form a protein complex that allows some proteins to function. The proteins that come together may be the same, closely related, or completely different.
But why do proteins have similar motifs? And if there are so many possible bond rotations, why do most proteins have only one identifiable form? This can be explained by a quantity known as Gibbs free energy, which is equal to the change in enthalpy minus the temperature times the entropy (ΔG=ΔH-TΔS). This quantity is commonly used to understand the spontaneity of reactions, but it also applies to protein folding. The free energy of a given state naturally minimizes through chemical reactions or conformational changes in folding. This concept helps explain protein structure. For example, in a secondary structure, hydrogen bonding releases energy, meaning that the change in enthalpy is negative. The entropy part of the equation is more complex. At first glance, it may seem that protein folding decreases entropy, which it does locally. However, for a reaction to happen spontaneously, the change in entropy should be positive to decrease the free energy in the system. To see why entropy increases as a protein folds, we must take into account the hydrophilic solution in which the protein exists–the cytoplasm of the cell. In an unfolded state, the protein restricts the movement of surrounding water molecules, lowering the solution’s overall entropy. When the protein folds, it reduces this restriction, allowing water molecules to move more freely, thus increasing the system’s entropy. Because protein folding has both a negative entropy (releases energy) and a positive entropy (maximizes the number of possible states for all of the molecules), the process occurs naturally.
For any given chain of amino acids, a protein can only fold in so many ways before it reaches the conformation with the lowest free energy. This suggests that, in theory, there should be a way to find the conformation of a protein from any amino acid sequence. Historically, scientists had to experimentally determine protein structures using methods such as X-ray crystallography, nuclear magnetic resonance, and other lab techniques. That is, until AlphaFold. Released about 5 years ago, AlphaFold is the first program to accurately predict protein shape from its primary structure, using artificial intelligence to determine the native state, or completely folded form. The AI analyses which protein motif is likely to be formed by amino acids—matching it to a domain in the protein database—by pairing every two amino acids and seeing to what extent mutations in that region correlate. Amino acids spatially close in a protein (not necessarily close in the primary sequence) are more likely to mutate together. Because the protein database has all of the protein domains, Alpha Fold can figure out how a protein folds.
The ability to predict protein shape is revolutionary because it makes determining the function of a particular gene easier. For example, if a protein is similar to a fiber, it is more likely to function as support for the cell. Or, if there's a site for ligands or other substrates to bind, then the protein may act as an enzyme or membrane protein. Additionally, this modeling has started being applied to other macromolecules, such as DNA and RNA in AlphaFold3. Structural research has advanced significantly because of AI and may even lead to novel ways to synthesize new treatments or medicines.
Citations:
Marcu, Ştefan-Bogdan, Sabin Tăbîrcă, and Mark Tangney. “An Overview of Alphafold’s
Breakthrough.” Frontiers, May 2, 2022. https://www.frontiersin.org/journals/artificial-
Skolnick J, Gao M, Zhou H, Singh S. AlphaFold 2: Why It Works and Its Implications for
Understanding the Relationships of Protein Sequence, Structure, and Function. J Chem
Inf Model. 2021 Oct 25;61(10):4827-4831. doi: 10.1021/acs.jcim.1c01114. Epub 2021 Sep
29. PMID: 34586808; PMCID: PMC8592092.
Comments