Author: Melinda Zhao
Editors: Hwi-On Lee, Junyu Zheng
Artist: Rachel Zhou
As research within the medical field expands and improves, discoveries are made daily, especially regarding cancer. Cancer remains one of the most formidable challenges facing humanity, ranked as the second-highest cause of death globally and accounting for 600,000 deaths annually in the US. Many people at some point in their lives have unfortunately either known of someone who was diagnosed with cancer, has been diagnosed themselves, or has at least heard of it.
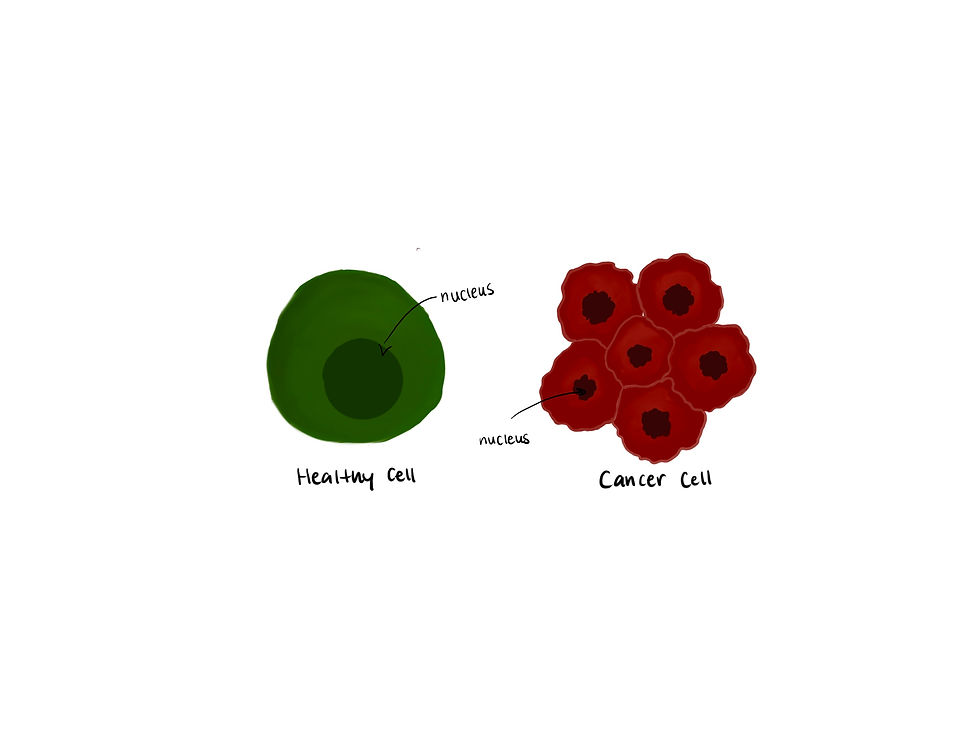
Cancer is defined by the uncontrollable growth of the body’s cells, which can spread to other parts of the body. Our body is made up of well over a million cells, and normally, it maintains the right balance of cells by controlling their division, multiplication, and through apoptosis, which is the programmed self-destruction of cells. However, cancer begins when genes mutate and create cancerous cells, which ignore these regulations and continue living. Since cancerous cells spread and replicate at a fast rate, it is imperative for them to be identified early on.
Healthcare providers diagnose cancer through various tests: blood tests, imaging tests, and biopsies. In breast cancer specifically, it is mostly diagnosed through a mammogram, which is an X-ray examination of the breast. However, mammograms are not flawless. They can take a long time to return a diagnosis and have the potential to produce false positives or negatives, leading to unnecessary stress or delayed treatment. Thus, this is where advancements in technology come into play.
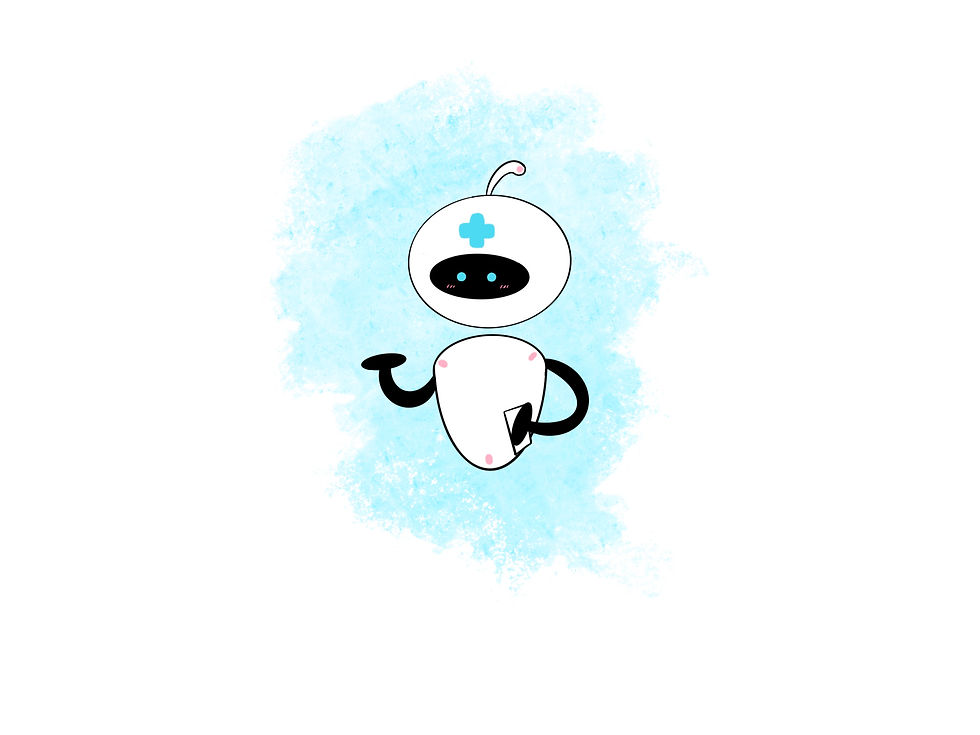
By analyzing a large dataset of thousands to millions of mammograms, AI software can create a system of maps to detect what a mammogram with cancer looks like. Users feed the model with images and run it through multiple training rounds using a chosen machine-learning algorithm. As the AI trains, it becomes more accurate in predicting what images are more likely to show cancerous activity. Lastly, users will test the AI using live data sets to see how well it performs.
Radiologists benefit from using AI to detect cancer by checking their reading of mammograms with the computer, identifying cancer earlier, and reducing false alarms. This can, in turn, reduce unnecessary follow-up testing as AI becomes a helping hand in confirming a diagnosis based on mammogram imaging. Researchers at MIT have recently developed an AI model specifically for ductal carcinoma in situ (DCIS), which is a type of hard-to-identify preinvasive tumor. Previously, researchers used expensive testing like multiplexed staining or single-cell RNA sequencing to detect the stage of DCIS. However, this new research uses machine learning to provide more information with improved accuracy without using these costly techniques. These researchers were able to design a model to detect nuanced changes within cell organization to find if the scan results could mean cancer development. Getting a more concrete diagnosis early reduces overtreatment toxicity, which refers to the harmful side effects of unnecessarily aggressive treatments, and also cuts down on further testing expenses. Additionally, AI tools such as image enhancement and de-noising can improve the quality of scanned images received, resulting in better viewing for radiologists and computers alike. AI can detect nuanced differences, estimate tumor size and shape, and identify hidden patterns among these scans.
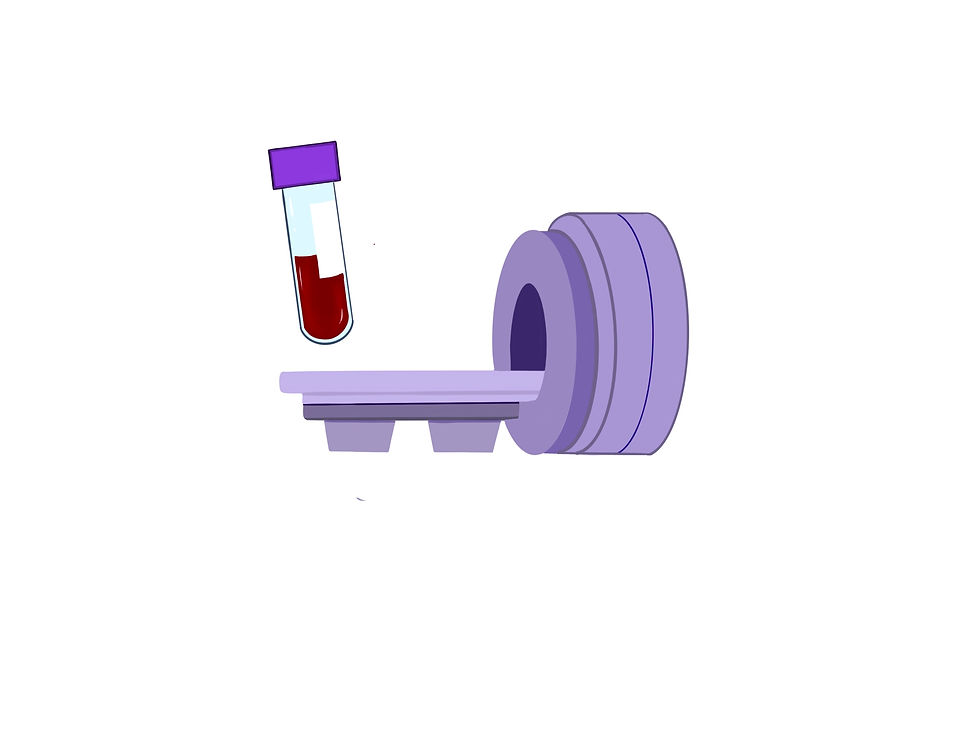
In the future, these models can be implemented in more than just breast cancer and include other types of cancer or just any scan interpretation by AI, like X-rays, CTs, MRIs, Ultrasound, and PET scans. Further advancements can enable AI to personalize treatment plans or specify the diagnosis based on individual genetic profiles. The possibilities of technology in medicine are endless, improving and transforming how we understand and combat diseases like cancer.
Citations:
“AI-Assisted Breast Cancer Screening May Reduce Unnecessary Testing.” Washington
University School of Medicine, medicine.wustl.edu/news/ai-assisted-breast-cancer-
screening-may-reduce-unnecessary-testing/. Accessed 18 Aug. 2024.
“AI in Breast Cancer Detection and Screening.” Breast Cancer Research Foundation,
www.bcrf.org/blog/ai-breast-cancer-detection-screening/. Accessed 18 Aug. 2024.
“AI Model Identifies Certain Breast Tumor Stages Likely to Progress to Invasive Cancer.”
Accessed 18 Aug. 2024.
“All Leading Causes of Death by Demographics.” National Safety Council,
Accessed 18 Aug. 2024.
“Artificial Intelligence in Breast Cancer Screening.” Breastcancer.org,
www.breastcancer.org/screening-testing/artificial-intelligence. Accessed 18 Aug. 2024.
“Breast Cancer Diagnosis and Treatment.” Mayo Clinic, www.mayoclinic.org/diseases-
conditions/breast-cancer/diagnosis-treatment/drc-20352475. Accessed 18 Aug. 2024.
“Breast Cancer Overview.” American Cancer Society, www.cancer.org/cancer/types/breast-
cancer/about/what-is-breast-cancer.html. Accessed 18 Aug. 2024.
“Cancer Overview.” Cleveland Clinic, my.clevelandclinic.org/health/diseases/12194-cancer.
Accessed 18 Aug. 2024.
“Causes of Death.” Our World in Data, ourworldindata.org/causes-of-death. Accessed 18
Aug. 2024.
“How Does AI Model Training Work?” Appian Blog, appian.com/blog/acp/ai/how-does-ai-
model-training-work. Accessed 18 Aug. 2024.
“Mammogram Procedure.” Johns Hopkins Medicine,
procedure. Accessed 18 Aug. 2024.
“What Is Cancer?” Cancer Research UK, www.cancerresearchuk.org/about-cancer/what-is-
cancer. Accessed 18 Aug. 2024.
“What Is Cancer?” National Cancer Institute, www.cancer.gov/about-
cancer/understanding/what-is-cancer. Accessed 18 Aug. 2024.
Comments